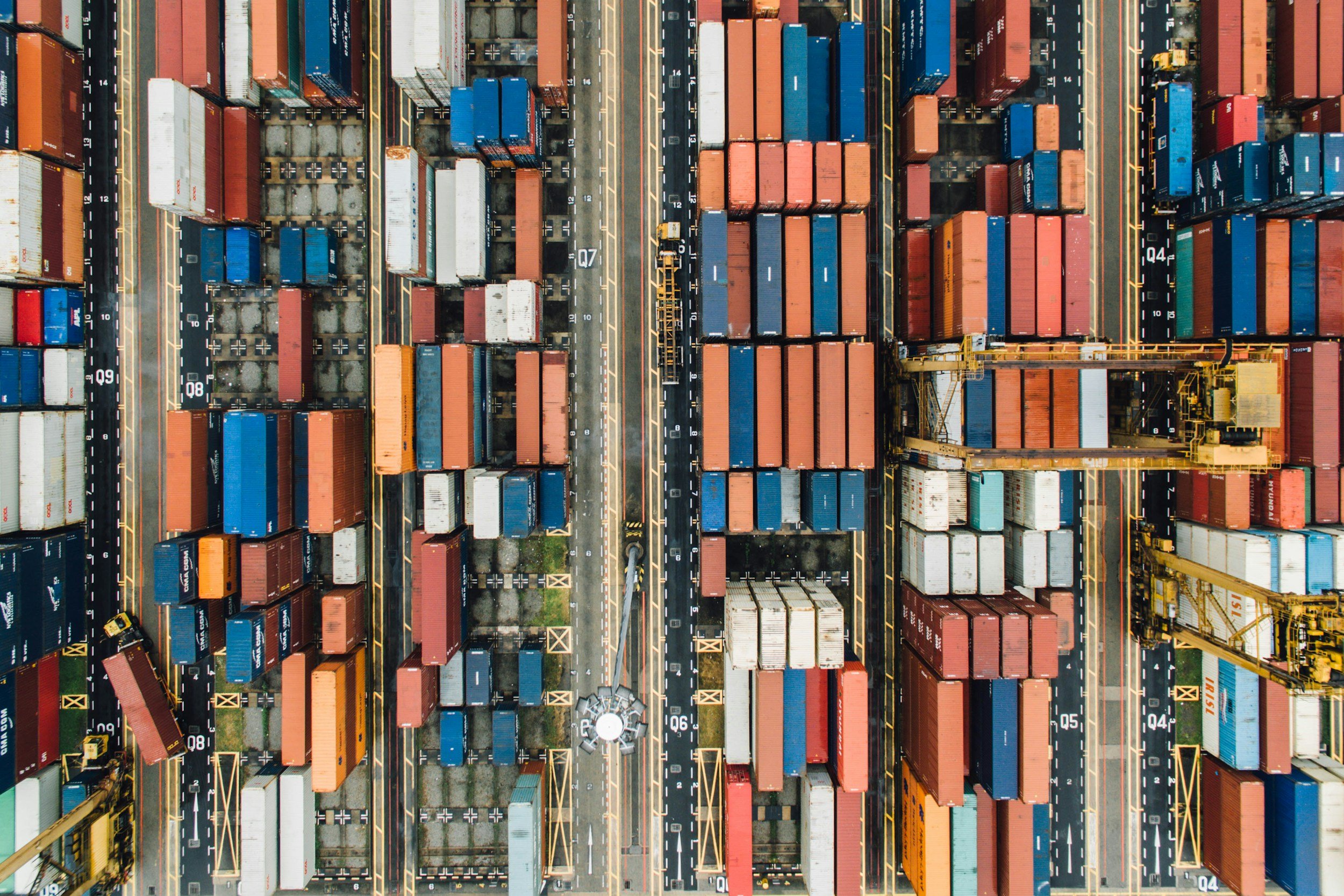
CASE STUDY
Automating Listing Classification with Machine Learning
The Smart Tagging feature was introduced to address the inefficiencies analysts faced when manually reviewing product listings. The key issue was the repetitive nature of reclassifying the duplicate irrelevant listings daily. By implementing a user-defined, open-box tagging system powered by machine learning, OpSec allowed analysts to create rules and automate classification based on accuracy scores. This resulted in substantial efficiency gains—up to 62%—and improved trust between the analysts and the system, with automation enabled once accuracy metrics were achieved.
Client: OpSec
Position: Dir. od UX
Duration: 6 Months
Problem:
Analysts were overwhelmed by the repetitive task of manually reclassifying product listings, with the Harvester unable to filter out previously reviewed data. This led to fatigue and inefficiency, while previous attempts at automation had failed due to a lack of transparency and trust.
Solution:
The Smart Tagging feature offered a transparent, "open-box" solution where analysts could create custom rules for automating the classification process. Analysts were empowered to refine and train the system, fostering trust and engagement—the tagging system allowed for both parent and child tags, providing granular control over the classification process. Automation was activated once accuracy thresholds were met, streamlining the daily workload.
Smart Tagging Workflow:
The Smart Tagging system was ine accordion-style interface, built using Bootstrap and Kendo UI, enabled deep customization.tegrated into a user-friendly HUD, allowing analysts to create and manage tagging rules with ease. Through visual aids such as iconography and blade systems, analysts could quickly review and adjust rules, ensuring accurate tagging of listings across global marketplaces. The accordion-style interface, built using Bootstrap and Kendo UI, enabled deep customization.
Initial KPI results point to efficiency gains in some cases as much as 62% at most, at minimum, 18%-24% in how day-to-day work was reviewed.
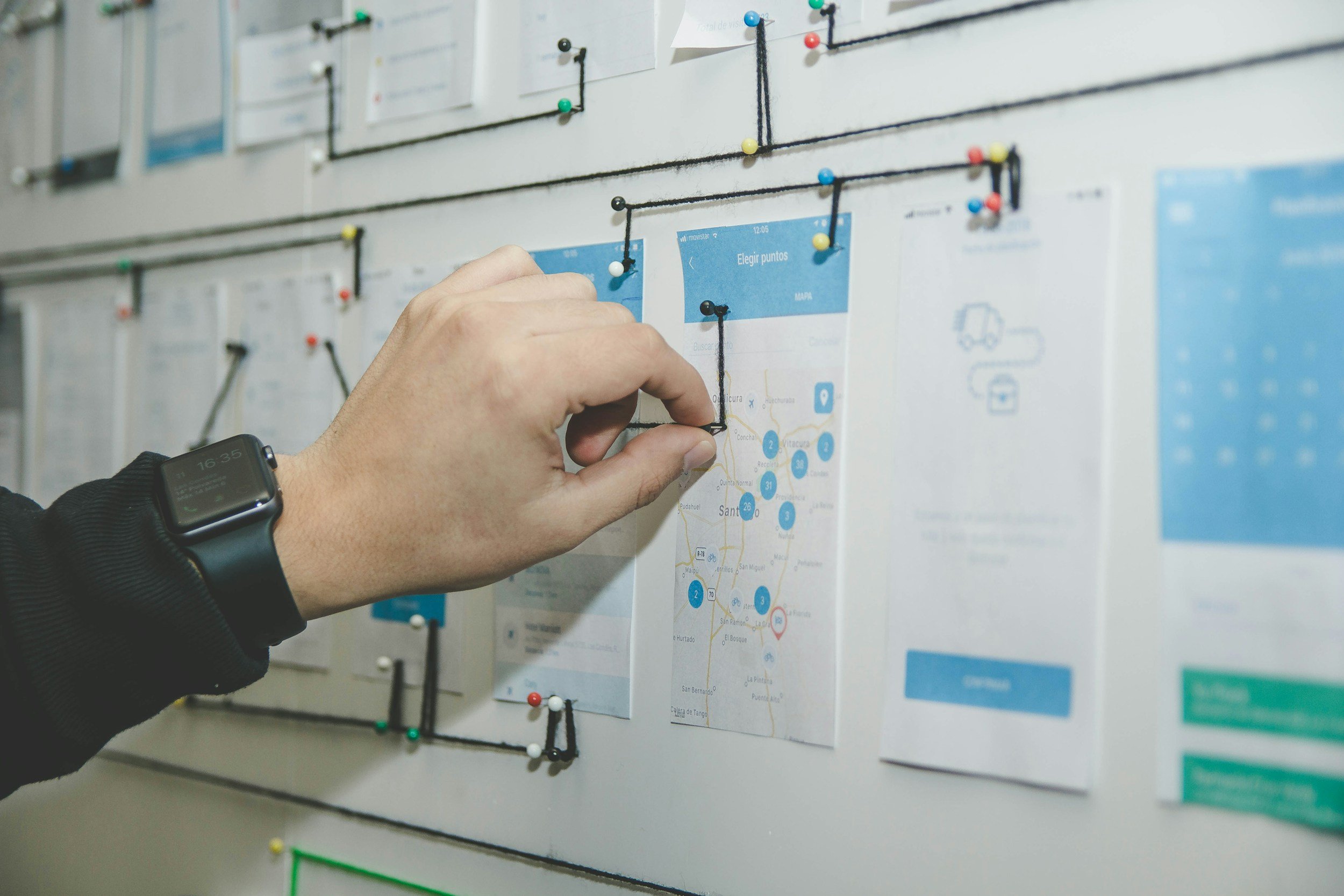
Machine Learning and Rule Overlap:
Machine learning played a crucial role in continuously refining the Smart Tagging process. Analysts could test rule-sets using real-time harvested data and adjust them as needed before full automation. Overlapping rule-sets provided analysts with additional insights, enhancing their decision-making abilities and increasing efficiency when processing large datasets.
Results:
Smart Tagging led to significant time savings, with analysts experiencing a 38% to 62% increase in efficiency. The transparent, customizable automation process improved trust between the product team and analysts, ultimately streamlining workflows and delivering higher-quality classification of product listings. As a result, OpSec analysts were better equipped to handle the daily influx of listings while maintaining high accuracy and effectiveness.
Launch the Figma prototype for the context menu navigation and iconography IA redesign.